Optimization AI data processing
Optimization AI data processing involves the utilization of ai models to enhance the process optimization of data sets. By integrating ai and machine learning techniques, ai can help in optimizing your data for better outcomes.
Whether it is big data or a specific type of data, ai process optimization can transform raw data into valuable insights. The data collection and data storage phases are crucial in ensuring that the data quality remains intact throughout the analysis process.
One of the key advantages of ai application in data science is its ability to handle large amounts of data efficiently. By employing generative ai and optimization techniques, ai excels in data transformation and data visualization.
The integration of ai into the data based data cleaning and data visualization processes can significantly enhance the data and provide better insights for decision-making.
Ai plays a crucial role in data classification and data based data to improve the overall data quality. Whether it is qualitative data or quantitative data, ai can also assist in the analysis process to derive meaningful conclusions.
The ultimate goal of ai process optimization is to ensure that businesses have access to better data for making informed decisions.
With the growing importance of data science and the increasing amount of data being generated, the role of ai in data processing will only continue to expand.
By employing ai algorithms and optimization techniques, organizations can streamline their data processing workflows and extract valuable insights from their data.
In conclusion, the integration of ai in the data processing workflow can lead to improved data quality and enhanced decision-making processes.
What is AI data processing?
AI data processing refers to the use of artificial intelligence technology to analyze, interpret, and manipulate data in an efficient and effective manner.
This technology leverages algorithms and machine learning techniques to automate data processing tasks and extract valuable insights from large datasets.
AI data processing involves the following key aspects:
- Advanced algorithms: AI utilizes advanced algorithms to process data quickly and accurately.
- Machine learning: By employing machine learning techniques, AI can continuously improve its data processing capabilities.
- Natural language processing: AI can understand and process human language, enabling it to analyze unstructured data such as text and speech.
Optimization AI data
Benefits of AI data processing
There are several benefits to using AI for data processing and optimization:
- Efficiency: AI can process large volumes of data at a much faster rate than humans, saving time and resources. This allows organizations to make quicker decisions based on real-time data.
- Accuracy: AI algorithms are designed to minimize errors and improve the accuracy of data analysis. This ensures that organizations can rely on the data insights generated by AI.
- Insights: By analyzing complex patterns and relationships in data, AI can uncover valuable insights that may not be apparent to human analysts. These insights can drive strategic decision-making and business growth.
- Automation: AI can automate repetitive data processing tasks, allowing organizations to focus on more strategic initiatives. This frees up human resources to work on higher-value tasks.
- Scalability: AI technology can scale up or down based on data processing needs, making it adaptable to changing business requirements. This scalability ensures that organizations can handle data processing efficiently as their data volumes fluctuate.
How AI optimizes data processing
AI optimizes data processing in several ways:
1. Data cleansing and normalization
One of the key challenges in data processing is ensuring that the data is clean and consistent. AI algorithms can automatically identify and correct errors in data, such as missing values or inconsistencies. By cleansing and normalizing the data, AI ensures that the data is accurate and reliable for analysis.
AI achieves data cleansing and normalization through techniques such as:
- Outlier detection: AI algorithms can identify outliers in data that may skew the analysis results.
- Data deduplication: AI can remove duplicate entries in datasets to ensure data accuracy.
- Standardization: AI can standardize data formats and structures to maintain consistency across different datasets.
2. Predictive analytics
AI can be used to perform predictive analytics, which involves forecasting future trends and outcomes based on historical data. By analyzing patterns and relationships in the data, AI algorithms can predict customer behavior, market trends, and other key variables that impact business performance.
Predictive analytics with AI involves the following key steps:
- Data preprocessing: AI preprocesses data to identify relevant features and prepare the data for analysis.
- Model training: AI trains predictive models using historical data to make accurate predictions.
- Model evaluation: AI evaluates the performance of predictive models to ensure their accuracy and effectiveness.
3. Personalization
AI enables organizations to personalize their products and services based on individual customer preferences and behavior. By analyzing customer data, AI can recommend personalized content, products, and offers that are tailored to each customer’s unique needs and preferences.
Personalization with AI involves techniques such as:
- Collaborative filtering: AI recommends products or services based on the preferences of similar customers.
- Content-based filtering: AI recommends products or services based on the attributes of the items and the user’s preferences.
- Contextual personalization: AI delivers personalized recommendations based on the user’s current context, such as location or time of day.
4. Anomaly detection
AI algorithms can detect anomalies or irregularities in data that may indicate potential fraud, errors, or security breaches. By flagging these anomalies in real-time, organizations can take proactive measures to mitigate risks and protect their data assets.
Anomaly detection with AI involves the following techniques:
- Unsupervised learning: AI uses unsupervised learning algorithms to detect anomalies without the need for labeled data.
- Threshold-based detection: AI sets thresholds to identify data points that deviate significantly from the norm.
- Behavioral analysis: AI analyzes user behavior patterns to detect abnormal activities that may indicate security threats.
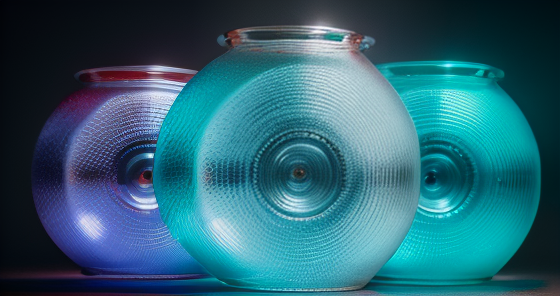
Challenges of AI data processing
While AI offers many benefits for data processing and optimization, there are also some challenges to consider:
- Data privacy: AI algorithms require access to large volumes of data, raising concerns about data privacy and security. Organizations must ensure that data privacy regulations are followed to protect sensitive information.
- Bias: AI algorithms may unintentionally perpetuate biases present in the data, leading to unfair outcomes or decisions. Organizations need to implement measures to mitigate bias in AI algorithms and ensure fair data processing.
- Interpretability: Some AI algorithms are complex and difficult to interpret, making it challenging to understand how decisions are made. Organizations must strive to improve the interpretability of AI systems to enhance trust and transparency.
Conclusion
AI technology is transforming the way organizations process and optimize data. By leveraging AI algorithms, organizations can improve efficiency, accuracy, and insights in their data processing activities.
While there are challenges to overcome, the benefits of AI data processing far outweigh the risks. As AI continues to evolve, we can expect to see even greater advancements in data processing and optimization in the future.
FAQ
1. What is AI data processing?
AI data processing refers to the use of artificial intelligence technology to analyze, interpret, and manipulate data in an efficient and effective manner.
This technology leverages algorithms and machine learning techniques to automate data processing tasks and extract valuable insights from large datasets.
2. What are the benefits of AI data processing?
- Efficiency: AI can process large volumes of data at a much faster rate than humans, saving time and resources.
- Accuracy: AI algorithms are designed to minimize errors and improve the accuracy of data analysis.
- Insights: By analyzing complex patterns and relationships in data, AI can uncover valuable insights that may not be apparent to human analysts.
- Automation: AI can automate repetitive data processing tasks, allowing organizations to focus on more strategic initiatives.
3. How does AI optimize data processing?
AI optimizes data processing through data cleansing and normalization, predictive analytics, and personalization.
By automatically identifying and correcting errors in data, forecasting future trends, and personalizing products and services based on customer preferences, AI enhances the efficiency and accuracy of data processing.
4. Can AI technology scale based on data processing needs?
Yes, AI technology can scale up or down based on data processing needs, making it adaptable to changing business requirements. This scalability ensures that organizations can efficiently process and analyze data as their needs evolve.